目录
摘要
实现残差模块
ResNet18, ResNet34
RseNet50、 RseNet101、 RseNet152、
摘要
ResNet(Residual Neural Network)由微软研究院的Kaiming He等四名华人提出,通过使用ResNet Unit成功训练出了152层的神经网络,并在ILSVRC2015比赛中取得冠军,在top5上的错误率为3.57%,同时参数量比VGGNet低,效果非常明显。
模型的创新点在于提出残差学习的思想,在网络中增加了直连通道,将原始输入信息直接传到后面的层中,如下图所示:
传统的卷积网络或者全连接网络在信息传递的时候或多或少会存在信息丢失,损耗等问题,同时还有导致梯度消失或者梯度爆炸,导致很深的网络无法训练。ResNet在一定程度上解决了这个问题,通过直接将输入信息绕道传到输出,保护信息的完整性,整个网络只需要学习输入、输出差别的那一部分,简化学习目标和难度。VGGNet和ResNet的对比如下图所示。ResNet最大的区别在于有很多的旁路将输入直接连接到后面的层,这种结构也被称为shortcut或者skip connections。
在ResNet网络结构中会用到两种残差模块,一种是以两个3*3的卷积网络串接在一起作为一个残差模块,另外一种是1*1、3*3、1*1的3个卷积网络串接在一起作为一个残差模块。如下图所示:
ResNet有不同的网络层数,比较常用的是18-layer,34-layer,50-layer,101-layer,152-layer。他们都是由上述的残差模块堆叠在一起实现的。 下图展示了不同的ResNet模型。
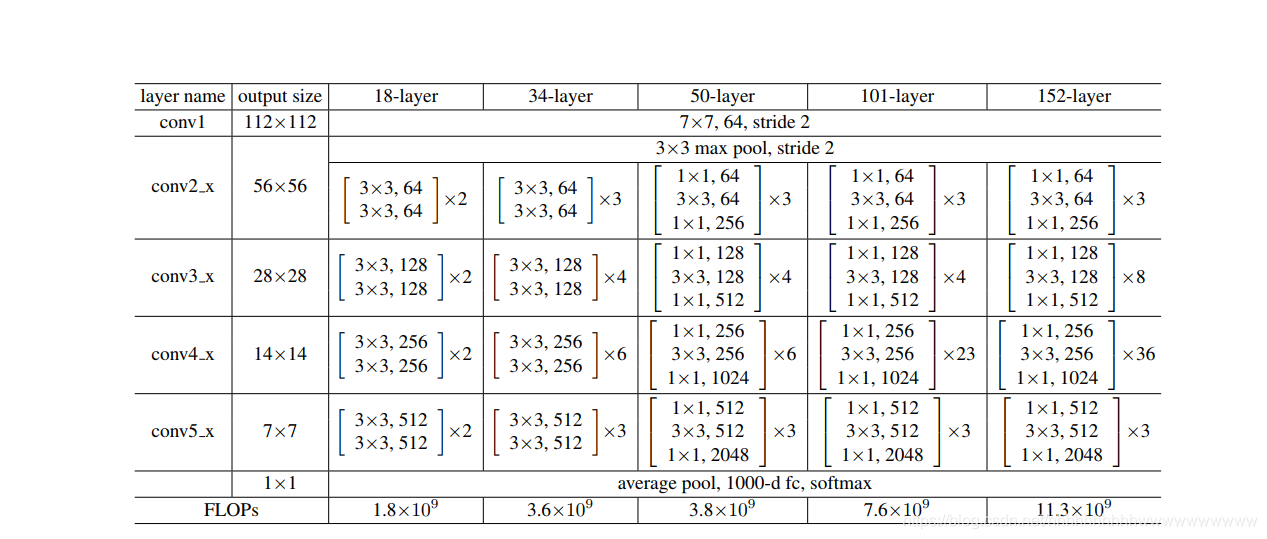
实现残差模块
第一个残差模块
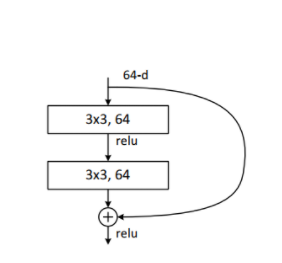
class ResidualBlock(nn.Module):
"""
实现子module: Residual Block
"""
def __init__(self, inchannel, outchannel, stride=1, shortcut=None):
super(ResidualBlock, self).__init__()
self.left = nn.Sequential(
nn.Conv2d(inchannel, outchannel, 3, stride, 1, bias=False),
nn.BatchNorm2d(outchannel),
nn.ReLU(inplace=True),
nn.Conv2d(outchannel, outchannel, 3, 1, 1, bias=False),
nn.BatchNorm2d(outchannel))
self.right = shortcut
def forward(self, x):
out = self.left(x)
residual = x if self.right is None else self.right(x)
out += residual
return F.relu(out)
第二个残差模块
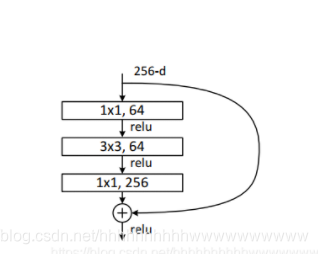
class Bottleneck(nn.Module):
def __init__(self,in_places,places, stride=1,downsampling=False, expansion = 4):
super(Bottleneck,self).__init__()
self.expansion = expansion
self.downsampling = downsampling
self.bottleneck = nn.Sequential(
nn.Conv2d(in_channels=in_places,out_channels=places,kernel_size=1,stride=1, bias=False),
nn.BatchNorm2d(places),
nn.ReLU(inplace=True),
nn.Conv2d(in_channels=places, out_channels=places, kernel_size=3, stride=stride, padding=1, bias=False),
nn.BatchNorm2d(places),
nn.ReLU(inplace=True),
nn.Conv2d(in_channels=places, out_channels=places*self.expansion, kernel_size=1, stride=1, bias=False),
nn.BatchNorm2d(places*self.expansion),
)
if self.downsampling:
self.downsample = nn.Sequential(
nn.Conv2d(in_channels=in_places, out_channels=places*self.expansion, kernel_size=1, stride=stride, bias=False),
nn.BatchNorm2d(places*self.expansion)
)
self.relu = nn.ReLU(inplace=True)
def forward(self, x):
residual = x
out = self.bottleneck(x)
if self.downsampling:
residual = self.downsample(x)
out += residual
out = self.relu(out)
return out
ResNet18, ResNet34
import torch
import torchvision
from torch import nn
from torch.nn import functional as F
from torchsummary import summary
class ResidualBlock(nn.Module):
"""
实现子module: Residual Block
"""
def __init__(self, inchannel, outchannel, stride=1, shortcut=None):
super(ResidualBlock, self).__init__()
self.left = nn.Sequential(
nn.Conv2d(inchannel, outchannel, 3, stride, 1, bias=False),
nn.BatchNorm2d(outchannel),
nn.ReLU(inplace=True),
nn.Conv2d(outchannel, outchannel, 3, 1, 1, bias=False),
nn.BatchNorm2d(outchannel)
)
self.right = shortcut
def forward(self, x):
out = self.left(x)
residual = x if self.right is None else self.right(x)
out += residual
return F.relu(out)
class ResNet(nn.Module):
"""
实现主module:ResNet34
ResNet34包含多个layer,每个layer又包含多个Residual block
用子module来实现Residual block,用_make_layer函数来实现layer
"""
def __init__(self, blocks, num_classes=1000):
super(ResNet, self).__init__()
self.model_name = 'resnet34'
# 前几层: 图像转换
self.pre = nn.Sequential(
nn.Conv2d(3, 64, 7, 2, 3, bias=False),
nn.BatchNorm2d(64),
nn.ReLU(inplace=True),
nn.MaxPool2d(3, 2, 1))
# 重复的layer,分别有3,4,6,3个residual block
self.layer1 = self._make_layer(64, 64, blocks[0])
self.layer2 = self._make_layer(64, 128, blocks[1], stride=2)
self.layer3 = self._make_layer(128, 256, blocks[2], stride=2)
self.layer4 = self._make_layer(256, 512, blocks[3], stride=2)
# 分类用的全连接
self.fc = nn.Linear(512, num_classes)
def _make_layer(self, inchannel, outchannel, block_num, stride=1):
"""
构建layer,包含多个residual block
"""
shortcut = nn.Sequential(
nn.Conv2d(inchannel, outchannel, 1, stride, bias=False),
nn.BatchNorm2d(outchannel),
nn.ReLU()
)
layers = []
layers.append(ResidualBlock(inchannel, outchannel, stride, shortcut))
for i in range(1, block_num):
layers.append(ResidualBlock(outchannel, outchannel))
return nn.Sequential(*layers)
def forward(self, x):
x = self.pre(x)
x = self.layer1(x)
x = self.layer2(x)
x = self.layer3(x)
x = self.layer4(x)
x = F.avg_pool2d(x, 7)
x = x.view(x.size(0), -1)
return self.fc(x)
def ResNet18():
return ResNet([2, 2, 2, 2])
def ResNet34():
return ResNet([3, 4, 6, 3])
if __name__ == '__main__':
device = torch.device("cuda:0" if torch.cuda.is_available() else "cpu")
model = ResNet34()
model.to(device)
summary(model, (3, 224, 224))
RseNet50、 RseNet101、 RseNet152、
import torch
import torch.nn as nn
import torchvision
import numpy as np
print("PyTorch Version: ",torch.__version__)
print("Torchvision Version: ",torchvision.__version__)
__all__ = ['ResNet50', 'ResNet101','ResNet152']
def Conv1(in_planes, places, stride=2):
return nn.Sequential(
nn.Conv2d(in_channels=in_planes,out_channels=places,kernel_size=7,stride=stride,padding=3, bias=False),
nn.BatchNorm2d(places),
nn.ReLU(inplace=True),
nn.MaxPool2d(kernel_size=3, stride=2, padding=1)
)
class Bottleneck(nn.Module):
def __init__(self,in_places,places, stride=1,downsampling=False, expansion = 4):
super(Bottleneck,self).__init__()
self.expansion = expansion
self.downsampling = downsampling
self.bottleneck = nn.Sequential(
nn.Conv2d(in_channels=in_places,out_channels=places,kernel_size=1,stride=1, bias=False),
nn.BatchNorm2d(places),
nn.ReLU(inplace=True),
nn.Conv2d(in_channels=places, out_channels=places, kernel_size=3, stride=stride, padding=1, bias=False),
nn.BatchNorm2d(places),
nn.ReLU(inplace=True),
nn.Conv2d(in_channels=places, out_channels=places*self.expansion, kernel_size=1, stride=1, bias=False),
nn.BatchNorm2d(places*self.expansion),
)
if self.downsampling:
self.downsample = nn.Sequential(
nn.Conv2d(in_channels=in_places, out_channels=places*self.expansion, kernel_size=1, stride=stride, bias=False),
nn.BatchNorm2d(places*self.expansion)
)
self.relu = nn.ReLU(inplace=True)
def forward(self, x):
residual = x
out = self.bottleneck(x)
if self.downsampling:
residual = self.downsample(x)
out += residual
out = self.relu(out)
return out
class ResNet(nn.Module):
def __init__(self,blocks, num_classes=1000, expansion = 4):
super(ResNet,self).__init__()
self.expansion = expansion
self.conv1 = Conv1(in_planes = 3, places= 64)
self.layer1 = self.make_layer(in_places = 64, places= 64, block=blocks[0], stride=1)
self.layer2 = self.make_layer(in_places = 256,places=128, block=blocks[1], stride=2)
self.layer3 = self.make_layer(in_places=512,places=256, block=blocks[2], stride=2)
self.layer4 = self.make_layer(in_places=1024,places=512, block=blocks[3], stride=2)
self.avgpool = nn.AvgPool2d(7, stride=1)
self.fc = nn.Linear(2048,num_classes)
for m in self.modules():
if isinstance(m, nn.Conv2d):
nn.init.kaiming_normal_(m.weight, mode='fan_out', nonlinearity='relu')
elif isinstance(m, nn.BatchNorm2d):
nn.init.constant_(m.weight, 1)
nn.init.constant_(m.bias, 0)
def make_layer(self, in_places, places, block, stride):
layers = []
layers.append(Bottleneck(in_places, places,stride, downsampling =True))
for i in range(1, block):
layers.append(Bottleneck(places*self.expansion, places))
return nn.Sequential(*layers)
def forward(self, x):
x = self.conv1(x)
x = self.layer1(x)
x = self.layer2(x)
x = self.layer3(x)
x = self.layer4(x)
x = self.avgpool(x)
x = x.view(x.size(0), -1)
x = self.fc(x)
return x
def ResNet50():
return ResNet([3, 4, 6, 3])
def ResNet101():
return ResNet([3, 4, 23, 3])
def ResNet152():
return ResNet([3, 8, 36, 3])
if __name__=='__main__':
#model = torchvision.models.resnet50()
model = ResNet50()
print(model)
input = torch.randn(1, 3, 224, 224)
out = model(input)
print(out.shape)
|